With some implications for the debate over assessing fiscal and monetary policies
Reader Brian writes:
DSGE’s aren’t the answer to everything, but I still find the microfoundations, careful treatment of expectations, etc. still attractive and, in my opinion, the best we have at the moment.
As somebody who has served on many dissertation committees where the dissertation involves cutting edge DSGEs (dynamic stochastic general equilibrium models), I can attest to the fact that such models can be very useful in providing insights into the workings of the macroeconomy, as well as the welfare implications associated differing policy regimes.
However, I think Brian’s observations highlight several misconceptions, and one important drawback of DSGEs. (An excellent review of the use of DSGEs in policy is provided by C. Tovar)
Misconceptions
Regarding the treatment of expectations, DSGEs usually incorporate model consistent expectations. However, ever since John Taylor’s pathbreaking work in the early 1990s [0], we have had model-consistent expectations imbedded in certain structural macroeconometric models. Hence, a DSGE is not necessary for operationalizing rational expectations.
Regarding microfoundations, if one examines the guts of the standard New Keynesian versions (including the one recently used by John Taylor [1]), one usually finds lots of ad hoc additions. Consumption is definitely not described by a simple Euler equation as implied by the pure rational expectations-life cycle hypothesis; usually there are some hand-to-mouth consumers floating around. Prices are not freely flexible; rather Calvo pricing is often assumed for tractability. Capital adjustment costs, and other frictions are often included as well. Why not leave these frictions out? Because, without them, it is well nigh impossible to replicate the impulse response functions of real world data. In other words, the bright line of microfoundations versus ad hoc functions is in fact pretty fuzzy.
(And from an international finance perspective, it’s troubling that the real exchange rate is usually linked one-for-one with the ratio of the marginal utilities of consumption, something that is as counterfactual as one can get. And don’t get me started on the risk premium gets introduced into these models, if indeed there is one.)
The Big Drawback
DSGEs (and their predecessors, RBCs) are models of the business cycle. As such, they focus on the deviations from trend. However, in order to predict where the economy will be in one year, given current conditions and policies, one needs to know what the trend is. In other words, extracting the cycle from the trend is critically important. This is a point that James Morley made in his “Emperor has no clothes” paper.
This issue has long been recognized in the policy community. From Camilo Tovar:
Econometricians often fail to be able to observe the theoretical concepts modeled (eg the output
gap). So a first question is: how to match the theoretical concepts in DSGE models with those of
the observed data? This is not trivial (and certainly the problem is not exclusive to these models).
In the DSGE literature the theoretical concepts have been captured not against specific data
figures (say GDP levels or inflation) but against filtered data (eg by using the Hodrick-Prescott
filter). Filtering decomposes the data into a cyclical component and a trend component. The
cyclical component is what is frequently fed into the model. By doing so the analysis focuses
on the business cycle frequencies, mainly because it is considered that DSGE models are
better suited to explain short-run rather than long-run cycles. However, filtering has important
implications (see discussion in Del Negro and Schorfheide (2003)). One is that, forecasting
stays out of the reach of DSGE models since the goal is to forecast actual rather than filtered
data. The second is that the dynamics obtained do not match those required by policy makers,
weakening the usefulness of DSGE models as policy tools. Alternatives often used are the
linear detrending and demeaning of variables, as well, as transforming variables so that they
are stationary around a balanced growth path. In this last case, the problem is that the trend is
often assumed to follow an exogenous stochastic process, which is imposed by the researcher.
In order to highlight the real-world complications involved in this issue, consider two popular cycle-trend extraction methods used in the “business”: the Hodrick-Prescott (HP) filter, and band pass (BP) filter. I apply the HP and BP filters over the 1967Q1-11Q1 sample, and HP filter to the 1967Q1-09Q1 sample, and plot the resulting cycle components in Figure 1.
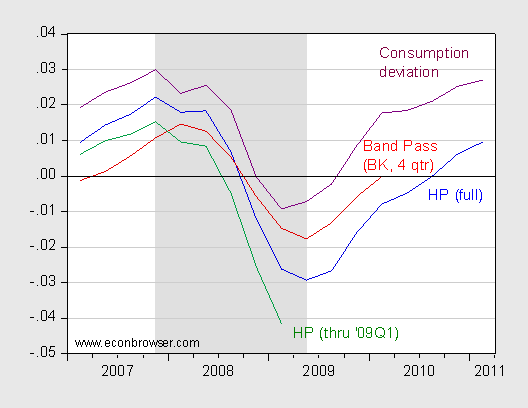
Figure 1: Log deviation from trend GDP, obtained using HP filter over entire sample, lambda=1600, estimated over 1967Q1-2011Q1 (blue), band pass filter with Baxter-King symmetric fixed length = 4 qtrs (red), HP filter over sample to 2009Q1 (green), and deviation from cointegrating relationship with services consumption (purple). NBER defined recession dates shaded gray. Source: BEA 2011Q1 3rd release, NBER, and author’s calculations.
Figure 1 demonstrates how the inferences regarding the output gap differ wildly depending on the filter used; and the sensitivity of the results — even for a given filter — to the endpoints (notice how the HP filter gives different output gaps for 2009Q1 depending on the sample).
Appealing to simple theories does not necessarily yield uncontroversial results. Figure 1 also includes the trend implied if one thinks GDP and consumption (here services only) are cointegrated. That approach, outlined by Cogley and Schaan (1995) following Cochrane (1994), implies that the economy was 2.7% above trend in 2011Q1.
The large scale macroeconometric models usually rely upon some sort of production function approach to calculating the trend. The implied output gaps from the statistical filter approach and the CBO’s version of the production function approach (described here) are displayed in Figure 2.
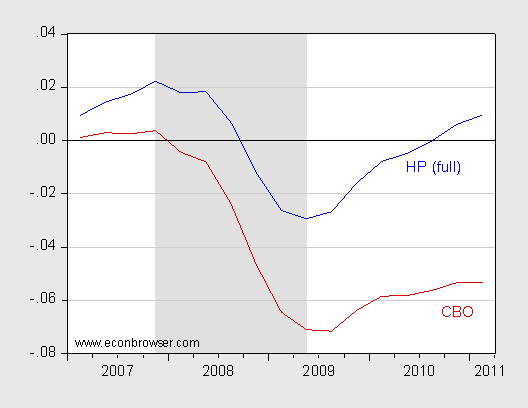
Figure 2: Log deviation from trend GDP, obtained using HP filter over entire sample, lambda=1600, estimated over 1967Q1-2011Q1 (blue), and log deviation from CBO’s potential GDP, January 2011 version (dark red). NBER defined recession dates shaded gray. Source: BEA 2011Q1 3rd release, CBO Budget and Economic Outlook (Jan. 2011), NBER, and author’s calculations.
For more on the scary things the HP filter can do, see T. Cogley, and J. Nason, 1995, “Effects of the Hodrick-Prescott filter on trend and difference stationary time series Implications for business cycle research,” Journal of Economic Dynamics and Control 19(1-2): 253-278. See also Simon van Norden on the use of these types of filters in current analysis.
[Update, 7/23 9:30am Pacific] An excellent, albeit technical, exposition of the issues involved by Gorodnichenko and Ng, here.
Concluding Thoughts
Much current macro research that I am familiar with is conducted using DSGE’s of one type or another. Very little research is conducted using large scale macroeconometric models. That doesn’t mean the results of those large scale macro models is useless, and that DSGE’s are inherently superior; one has to keep in mind the imperatives of academia are different from those pertaining to the policy world. And there are many different sets of models that have differing strengths vis a vis the two categories. Time series models might do better in forecasting. Small macroeconometric models can provide insights into the way the economy works, without necessarily being better at prediction. In general, the best type of model depends upon the question at hand; this depends not only on the model characteristics, but also the constraints imposed when faced with noisy and limited real-world (real-time) data.
Or, as my parents used to tell me ad nauseum, “new is not necessarily better” (roughly translated).
More on detrending here: [2] [3] [4]. More on differing output gaps here: [5] [6] [7]. And a DSGE with relatively large multipliers (compared against Smets-Wouter) discussed here, while a comparison of DSGEs is discussed here.
Menzie, thanks for this post. It gives me a lot to chew on.
I apologize in advance if this is a dumb question (I’m just a student).
Regarding the microfoundations of DSGE models:
If a utility function can only be aggregated under certain conditions, and if those conditions are not met in a particular DSGE model, how can that one agent be “representative” of the macro economy given that this agent’s preferences don’t meet the conditions necessary for aggregation?
The whole “microfoundations” debate seems misplaced to me. Take, for example, production. The standard assumption seems to be of firms exchanging factors (labor, capital) for finished products at a rate determined by available technology and resource constraints. Where, for example, is the place in the model that accounts for the fact that firms generally have positive cash conversion cycles and therefore must finance working capital in order to continue operations? DSGE models assume away the need for finance and then we wonder why the impact of a breakdown in the financial system can’t be explained by the model.
Michael: Good question; I’m not an expert, but here’s my take. If all agents are identical (with identical wealth), then one can aggregate. The introduction of rule-of-thumb consumers is a bit ad hoc, but since they simply consume all their income, then one doesn’t need to worry about how aggregation affects them.
When the optimizing agents themselves exhibit heterogeneity in behavior or wealth, then things get much more complicated (i.e., much easier to call for than to implement). Others can chime in on the recent work here; limited participation models seem particularly of interest to me.
RueTheDay: I don’t completely disagree, but as I say, there is no model for all questions, and the assumptions of perfect capital markets might be useful to gain some insights, especially when the long horizon is of interest.
Menzie my experience is that perfect capital markets never exist. And international markets are a mixed martial arts contest where if you’re not cheating you’re not competing.
Is the assumption then that those imperfections somehow pretty much cancel each other out over the long term? My assumption is that over the ‘longterm’ the cumulative effect of these imperfections leaves one with a real world far from what was any models anticipated.
Let me preface the following acerbic-tinged remarks by upfront appreciation for this blog and the thought it provokes. No small compliment to the hosts.
“… one has to keep in mind the imperatives of academia are different from those pertaining to the policy world. … Time series models might do better in forecasting. Small macroeconometric models can provide insights into the way the economy works, without necessarily being better at prediction.”
What are the imperatives of the academic world? Of the policy world? And what about the real world – a far broader world than policy Washington or scientific academia – and manifestly the one the greats were concerned with? Adam Smith, Ricardo, JS Mill, Marx, Keynes, Schumpeter, Friedman … all said and done were they not precisely concerned with the real world? Of course they were, and they would not have stood still for this false pedagogy for one moment.
Exactly whom are you kidding if your model can’t predict? We get insight into the way the economy works from a model that can’t predict? What kind of science is this? Name me one insight into the real world worthy of anyone’s attention – in any of the sciences! – if that insight is not replicable (forecastable) in a scientific sense? This is delusion, Menzie. Indeed there is a value to models not necessarily better at prediction. Future researchers need not go down that road.
JBH: What do you do if in a historical simulation, the RMSE from one model is 1% smaller than that of another model? Do you blindly pick the model with a 1% smaller RMSE?
@JBH:
What do you mean with “can’t predict”? Menzie said that one model wasn’t necessarily better at prediction than the other model. If your bar is that a model needs to exactly predict reality w/o any range of uncertainty then you have a grave misunderstanding about science and what models and their purpose are.
Models are always based on simplifications and idealizations. What kind of science is it? It’s any kind of science, whether it’s physics, neuro sciences, or economy. No model can exactly predict the behavior of the object of study. The only model able to achieve that would be an exact copy of the object of study itself, an exact copy of nature, the brain, or the total of all economy on Earth.
JBH Models can have different purposes beyond just predicting. Sometimes you build an econometric model to better test for and understand the relationship between variables. A model can be very good at making near term predictions, but lousy at revealing longer term structural relationships. Econometrics is not only about making forecasts. And even if all you care about is making a forecast, you still need to have some kind of model in mind that is in some way informed by theory; and you need to design tests for that structural theory. Without some kind of theory in mind you sacrifice model parsimony, and that hurts out-of-sample fits even if it improves in-sample fits.
The problems of non linearity homogeneity and transitivity, and non linearity mathematics are not confined to economics or finance,Black Sholes options (and its axiomatic inventories of volatility) but to physics as well.
I found interesting to read this article as a testimony
“According to most cosmologists, there is nothing special about us as observers of the universe. Still, some theories shirk this so-called Copernican principle, suggesting that the universe is not homogeneous”
The rest may be read through this thread.
http://physics.aps.org/synopsis-for/10.1103/PhysRevLett.107.041301
The only problems with “the best that we have” is that we are never tempted to question the best that we have. Many thanks to M. Chinn and J.Hamilton to open the door for breathing.
One can aggregate under very weak assumptions on the utility function with complete contingent claims markets, see Duffie’s “Dynamic Asset Pricing Theory”, section 1E and 10F (second edition).
All:
Let us not dismiss JBH so lightly. Remember, this discussion is inextricably tied to a very real world question: did the ARRA improve social welfare? Economists address this kind of question using social welfare analysis, which is a mix of both positive and normative. The positive part attempts to estimate underlying parameters of utility functions using real world data. The normative part assumes certain aggregation(s) of individual utility functions to produce an index of social welfare. But the persuasive force of welfare analysis depends on the validity of the positive analysis. If the positive analysis does not fit the real world data very well, then the normative analysis is not very informative. Is there a study (or review) that quantifies the predictive validity of DSGEs? I get that no model will predict perfectly, but just how imperfect are the DSGEs? If the answer is that DSGEs do a poor job of predicting, then they cannot be a useful starting point for answering questions like: did the ARRA improve social welfare?
Adam P: Think about what “complete contingent claims markets” means…
MarkOhio: I don’t know of anybody who’s done a horse race over time; that would be difficult to do given the variety of models. A comparison over the 2007-09 recession is conducted by Volker Wieland. But one of the key issues is that the forecasts are highly dependent upon some of the auxilary assumptions like the detrending method, as discussed in the Gorodnichenko-Ng paper.
Let me preface this with my oversense of the field of economics. As MDs take an oath to help people with life, and the President swears an oath to the Constitution, I believe the lodestone of economics is growing society’s material standard of living. Adhering to tried and true scientific methodology is necessary in this endeavor. Friedman says a theory should be judged by the accuracy of its predictions. I have thought long and hard about this, and because Freidman’s notion captures how science actually works I agree with him. As well, the reason for which a model is constructed and the purpose for which it is being used is crucial, which is why I take care to state upfront what I think the lodestone should and must be.
Menzie. I try not to do anything blindly. To me the sine qua non is: does the model (theory) predict in real time. That said, the answer to your question is an all too obvious no I wouldn’t go by RMSE.
Rootless Whichever model predicts better is the one science must go with. Setting up a strawman such as “exactly predict reality w/o any range of uncertainty” is Procrustean language that does not really work for me or others. The stuff we are discussing is Darwinian survival, and the better-predicting model will tend to displace its less robust competitors over time, another way of saying what I already said. (And that said, please let me applaud your engaging here with your thoughts though they be different than mine.)
Slugs I suggest you cogitate some on what you are saying. (a) What does it mean to better test for and understand the relationship between variables? By what criterion do you know you better understand? I repeat, by what criterion? Read or reread Friedman’s The Methodology of Positive Economics. In the real world – the only arena that counts – just as all foxes end up at the furriers, all hypotheses, models, and economic theories eventually have their final judgment day at the hands of Prediction. (b) You are quite correct on the near-term vs. longer-term. Nonetheless it does not naysay that there must be some criterion to separate out the superior “longer term structural relationships” from their genetically inferior longer term peers. You by now know what I believe that criterion must be. Other criteria are left in the dust (though I am infinitely open to any specific you believe might be superior). (c) Come again about econometrics not being mostly (I carefully back away from your word “only”) about making forecasts? Or come again about econometrics not being about statistically verifying a posited causal connection between variables, which the summary statistics of econometrics will at the end of the day in an Emperor-has-no-clothes way reduce to adequate predictive ability or absence thereof. Note emphasis on at the end of the day. (d) As far as models informed by theory go, we all know about the Swiss-cheese-size holes of received macroeconomic theory through which you can drive eighteen wheelers. To reference Freidman once again: “The construction of hypotheses is a creative act of inspiration, intuition, invention, its essence is the vision of something new in familiar material.” This statement resonates with successful entrepreneurs, theory that has not passed muster in terms predictive prowess does not.
MarkOhio You see the light I am trying to shed. Yours gives me hope others will see it too. “If the positive analysis does not fit the real world data very well, then the normative analysis is not very informative.” Precisely and cogently said! Let me align the planets here. Economics is the profession charged with telling us how to grow GDP. Hard factual data show that in 2007 the entire profession – but for a few – missed completely the greatest event since the Depression. How can anyone then have confidence in the profession’s model policy pronouncements? Romer said $787 billion fiscal stimulus would ensure no worse than 8%, yet the unemployment rate went to 10%. This was a big prediction; it was wrong; and you have to explain that. It is not her fault. Let me end with a forecast hewed from the whetstone of many years of predictive performance: Come November 2012 the unemployment rate will be higher (!) than it is now because real GDP will grow more slowly than 2½%, as the inventory impetus has ended and the economy is being impeded by forces conventional macroeconomic theory does not adequately recognize. Celestial dome thinking trumps model RMSE at critical junctures like this … my deepest point of all.
JBH Romer said $787 billion fiscal stimulus would ensure no worse than 8%, yet the unemployment rate went to 10%. This was a big prediction; it was wrong; and you have to explain that.
Romer also said that the economy would turn around in June 2009. That prediction was right. The Romer paper was written in Dec 2008 when just about everyone underestimated how bad the economy actually was at the time. I’m not sure how that gets interpreted as getting the basic modeling wrong.
So in your world an atheoretical simple VAR with error terms that are composites of other error terms would be a superior economic model relative to a structural VAR even though the atheoretical VAR has no economic content. Is that how you see things?
Menzie, you said: “I’m not an expert, but here’s my take. If all agents are identical (with identical wealth), then one can aggregate.”
Think about what “all agents are identical” means.
As it is I think I have a pretty good idea what complete markets means. Since one of my general exams was financial economics and most macroeconomists know very little asset pricing theory I might even count myself an expert, at least in this conversation.
Since you brought up identical agents as a sufficient condition I assumed we were talking about simple sufficient conditions and not realistic ones.
Slugs At the beginning of 2009 when the CEA made its estimate, there was a known relationship between quarterly changes in the unemployment rate and real GDP growth from the data set of the prior expansion. One must get one’s hands dirty with the data to quantify this relationship. It was important not to take the estimation process back too far because the 2000-2007 period was the margin. At the very least you had to weigh recent quarters more than old ones. At the time of Rohmer’s forecast: (a) Dec 2008 unemployment was known to be 7.2%, and (b) the consensus expected -3.3, -0.8, 1.2, and 2.0% for GDP growth across the four quarters of 2009. Given the extant relationship between GDP (contemporaneous and lagged 1) and u – and using the consensus forecast for the explanatory variable – it was a no brainer to predict u of 9.2% for Q4:2009. It is vital to understand that the consensus (and everyone else in Western Civilization) knew that fiscal stimulus on the order of $1T was coming and was already incorporated into the consensus forecast. If you knew what you were doing you didn’t have to interpolate CBO, CEA, House and Senate stuff, you could cut right to the quick.
The consensus projection for Dec 2009 was 8.6%. Many consensus forecasters do not grasp, especially in times of great change, the importance of the methodological points I make here. Even so the 8.6% consensus projection – and the 9.2% estimate based on the structural and methodological view above – were far better the CEA’s official projection. And doable in real time. The actual turned out to be even higher at 10%.
As for your final comment, nothing I say or imply is in the least atheoretical. Mostly this is about methodology, though of course admixed with the way the universe works. This is a deep well. So let me tie a Gordian knot on the rope we use to pull the bucket up. The real world has neither time nor patience for theory that doesn’t work. Nor for forecasts that do not.
JBH Slugs At the beginning of 2009 when the CEA made its estimate
Except we weren’t talking about the CEA estimate. You were the one who brought up the Romer-Bernstein paper and that was written in Nov/Dec 2008.
At the time of Rohmer’s forecast: (a) Dec 2008 unemployment was known to be 7.2%
Well, no. The December unemployment rate did end up being 7.3%, but that number did not come out until after their paper was written. At the time they wrote their paper the known unemployment rate was 6.6% in Oct 2008 and 6.8% in Nov 2008.
It is vital to understand that the consensus (and everyone else in Western Civilization) knew that fiscal stimulus on the order of $1T was coming and was already incorporated into the consensus forecast.
Except of course that it never happened. I would agree that anyone with sense knew that the stimulus had to be well north of $1T and that Romer’s own calculations suggested something like $1.3T-$1.4T. But many people didn’t expect the GOP to be as stupid as they were nor did people expect Obama to be such an incompetent negotiator, nor did anyone expect the Minnesota Senate race (giving the Dems their 60th vote) to drag out for 6 months. So what we actually got was a weak stimulus package that was too little, too late.
Getting back to the original point. We all want models that forecast well; but we also want models that provide structural insights. A model can be very good at making short-run forecasts, yet utterly devoid of any economic content or meaning. And getting to Menzie’s point, teasing out trends (stochastic or deterministic) can be a very tricky business. And there is no guarantee that one model will work well across all time horizons. When you make a forecast you usually don’t just want to rely upon an unexplained time series relationship. Usually you would like to make sure the forecast is consistent with some structural interpretation.
2slug: If your structural model doesn’t provide a good forecast, that is often a sign that you need to continue working on your theory.
Adam P: I have thought long and hard about what it means to say “all agents are identical”. Well, I did say there are limitations associated with most of the DSGE’s out there. Heterogeneity in a meaningful sense is hard to incorporate as it makes the solution for equilibrium much more difficult (to say the least).
Let me chime in here in defense of JBH.
Start by thinking about available macroeconomic data. If we use quarterly data for the last 40 years, that gives us 160 observations. Before you say you have more data than that, ask yourself if you are confident that today’s economy is similar enough to the economy of 1950 to make that data relevant.
Economic time series are often somewhat collinear, so that is going to hurt when you estimate a statistical model. And what’s so magical about the quarterly frequency? What you’re really interested in are business-cycle fluctuations, which usually last a year or two. According to the NBER, there have been 12 business cycle peaks over the last 40 years, so you really only have 12 observations of the phenomena you’re interested in.
Now consider what happens when you estimate a statistical model with this limited data. The most general statistical model, which incorporates no economic theory whatsoever, is the Vector Auto Regression (VAR). A VAR(4) regresses each variable on 4 lags of itself and 4 lags of each of the other variables in the model. If N is the number of variables, that means you have 4*N*N coefficients. You also have N*(N+1)/2 parameters in the variance-covariance matrix to estimate. So for N = 4, you have 74 parameters to estimate. For N = 5, 115 parameters. For N = 6, 166 parameters.
The exploding number of parameters is why adding an additional variable to a small VAR usually decreases both its forecast accuracy and the precision of the individual coefficient estimates. Only if the new variable has a great deal of explanatory power does it hurt more than it helps.
On the other hand, if you put correct (or approximately correct) restrictions on the model parameters, precision and forecasts tend to improve, as the restrictions effectively limit the number of parameters that are being estimated. Once source of (hopefully correct) restrictions is economic theory.
You can also put “Bayesian” restrictions on the parameters. The Bayesian VAR (BVAR) is a kind of ridge regression that reduces the effective number of parameters estimated by biasing them in a particular direction. In the original BVAR work by Litterman and Sims, the bias was towards modeling the variables as independent random walks. This is usually the default direction in statistical software packages that estimate BVARs. Some packages also allow you to specify additional restrictions as well. But I want to point out that the default direction is completely atheoretic.
If you estimate a reasonable BVAR for macroeconomic variables, what you’ll typically find is that
(i) it forecasts much better than an unrestricted VAR,
(ii) it forecasts at least as well (usually better) than a VAR with restrictions implied by an economic theory model, and
(iii) adding theory-implied restrictions to the BVAR doesn’t noticeably improve its forecasting ability.
So there’s no statistical reason to believe the theory. Macroeconomic theories are just stories. They can give you “structural insights” but those insights aren’t really any more valuable than the ones you get from fortune tellers or phrenologists. They are used in the private sector to help persuade clients to part with their money. In the public sector they provide political cover for whatever the policy maker was going to do anyway. And in the academic world, mathematically challenging theories are used to disqualify those who can’t do the math when making publication and tenure decisions.
The one thing macro theory doesn’t do is teach us anything about the actual economy.
There are a few things we really know about macro economies. One is that, as Friedman said, inflation is a monetary phenomena. Another is that market economies work better than planned ones. We believe both of these assertions not because they fit a theory, but because we have seen them demonstrated time and again in the real world. The only “sort-of” macro theory I can think of offhand that is self-evidently true is Ricardo’s explanation of Comparative Advantage.